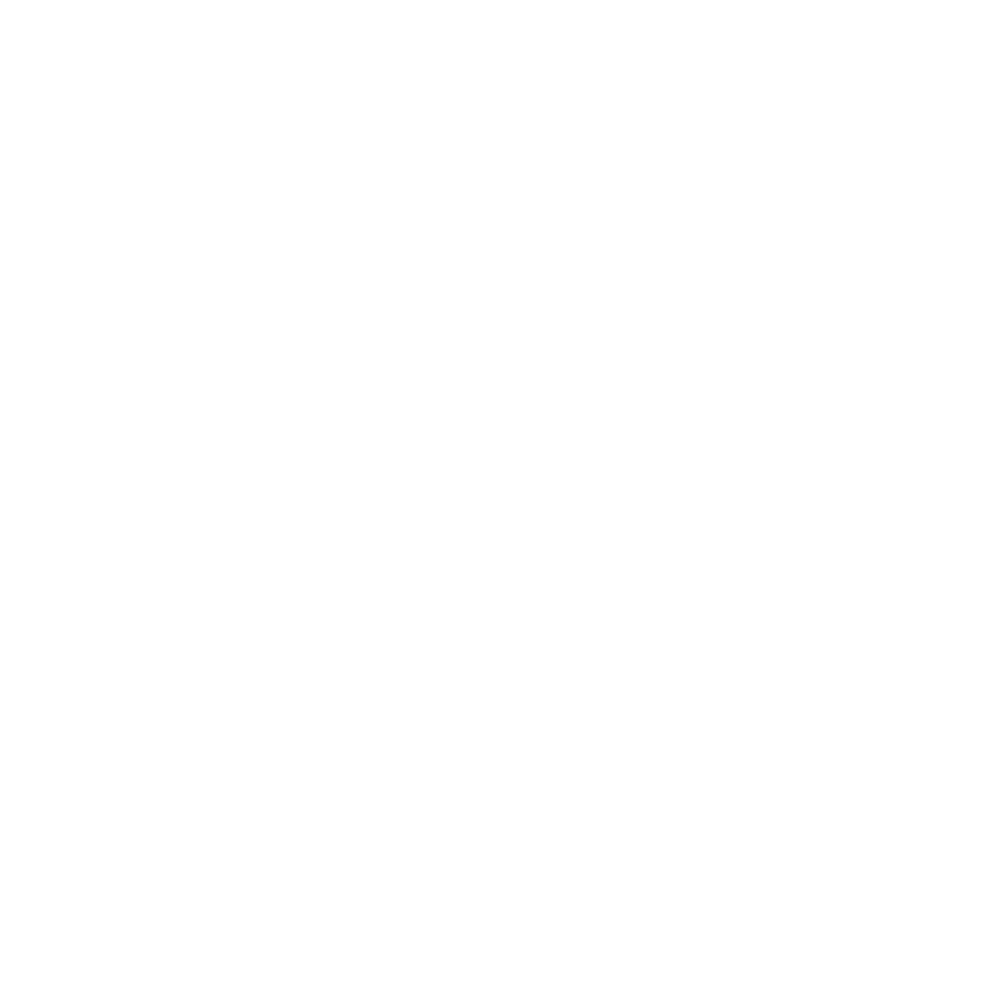
Reinforcement Learning on Agroecology
Shannon Brownlee * , Jordan Cahoon * , Aryan Gulati , Leslie Moreno , Megan Friedenberg , Colin Ho , Felix Chen
* Project Lead
Industrial agriculture is unsustainable and destroys local ecosystems. An alternative approach is to instead plant crops within existing microclimates instead of destroying them entirely. This approach has seen success on small scales, such as a Wisconsin farmer successfully growing his crops in a local forest. However, microclimates are very different from one another, and that Wisconsin farmer would have no idea how to succeed in growing crops in a Californian forest, for example. There is no universal method for doing things. CAIS++ students collaborated with Professor Raghavan’s lab to construct a reinforcement learning system using a blackbox environment that can simulate any arbitrary microclimate. Our mission is to model any given microclimate and find the optimal policies for growing crops in that microclimate.