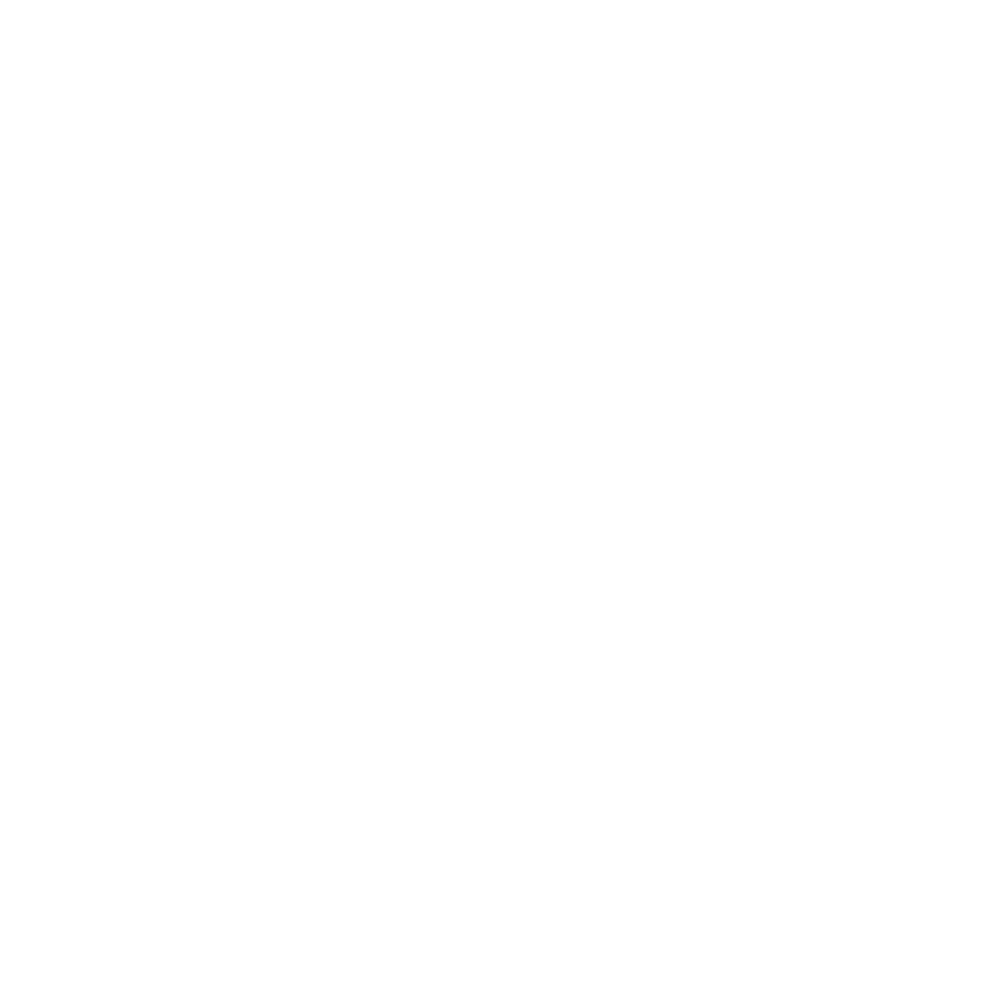
Multimodal Speech Recognition for Language-Guided Embodied Agents (2.0)
Allen Chang * , Xiaoyuan Zhu , Aarav Monga , Seoho Ahn , Tejas Srinivasan * , Jesse Thomason *
* Project Lead * Project Advisor
Benchmarks for language-guided embodied agents typically assume text-based instructions, but deployed agents will encounter spoken instructions. While Automatic Speech Recognition (ASR) models can bridge the input gap, erroneous ASR transcripts can hurt the agents' ability to complete tasks. In this work, we propose training a multimodal ASR model to reduce errors in transcribing spoken instructions by considering the accompanying visual context. We train our model on a dataset of spoken instructions, synthesized from the ALFRED task completion dataset, where we simulate acoustic noise by systematically masking spoken words. We find that utilizing visual observations facilitates masked word recovery, with multimodal ASR models recovering up to 30% more masked words than unimodal baselines. We also find that a text-trained embodied agent successfully completes tasks more often by following transcribed instructions from multimodal ASR models.